Energy company

Case
Building a prototype predictive model to forecast solar panel electricity generation
AI, ML and Predictive Analytics
Customer
Industry
Energetics
Scale
≥ 1000 employees
– Build a predictive model.
– Automate the prediction process.
– Decrease financial losses due to forecast errors.
– Provide the client with a detailed report on model accuracy and potential ways to increase it.
The customer provided us with the data they used to make the forecast, namely hourly data on electricity generation and daily temperature data.
To improve the accuracy of the predictive model, we enriched it with regional weather readings and data from various sources, including open ones. This allowed us to recognise and utilise hidden data patterns.
Based on the data analysis, we built models utilising several different architectures:
– decision trees,
– neural networks,
– metric-based algorithms.
We discovered the features that influence the forecast result the most, built a prototype predictive model, analyzed viability and means to implement the ML model to solve the problem, as well as the ways to improve it, and provided the client with the respective detailed report.
AM-BITS team achieved 84% model accuracy (on data from March to October 2021).
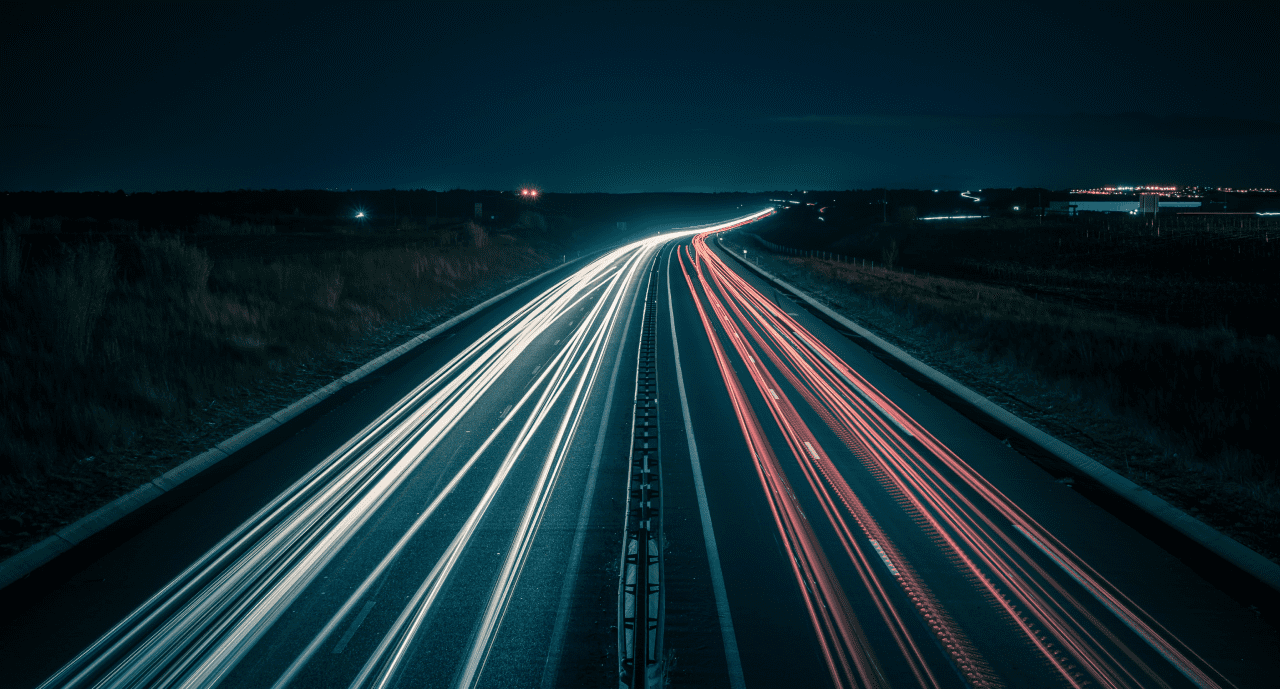
Would you like to see
the full case study?
Fill out the form and we will
contact you right away